The Impact of AI in Business Analytics: Challenges and Opportunities
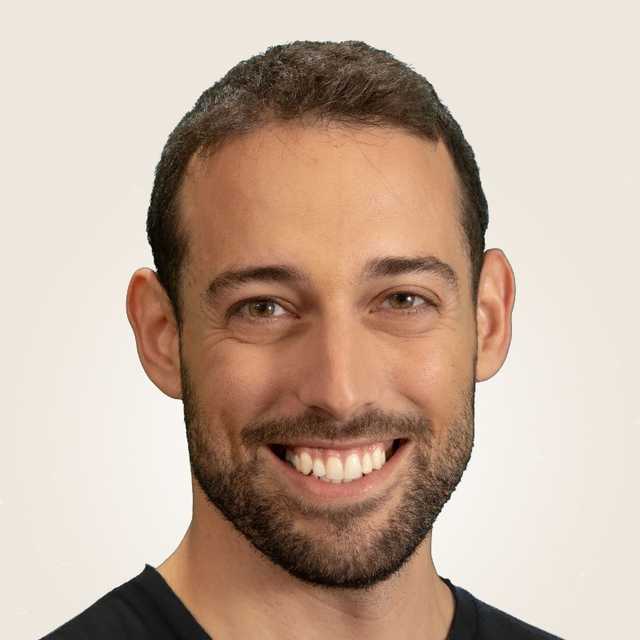
Senior Data Analyst
In 2024, AI is poised to transform how people and products work. Leaders today must have a balanced understanding of AI’s potential and limitations if they wish to effectively make use of this new technology.
In this article, we will explore AI’s transformational role in business analytics, its potential impact as well as the present challenges. business can expect to face as they attempt to fully leverage this technology for data analysis and decision-making.
Read on to learn about the power of tools like ChatGPT for data analytics and how to avoid the common pitfalls on the path to AI powered analytics.
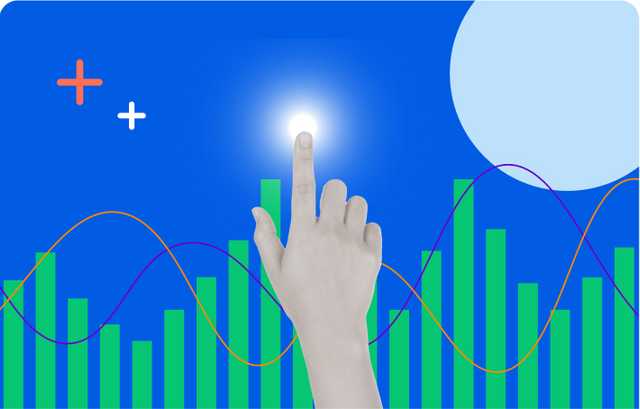
Introduction to AI in Business Analytics
In today's fast-paced, highly competitive business landscape, data analytics is essential for strategic decision-making. The advent of artificial intelligence (AI) has revolutionized the way businesses leverage data to gain insights and make informed decisions at every level of the organization. In business analytics especially, AI has been a game-changer, empowering business users to more efficiently uncover hidden patterns, identify growth opportunities, and optimize their operations.
Over the past few years, AI has evolved rapidly. One of the most exciting developments is the rise of Generative AI (GAI) tools like ChatGPT. ChatGPT is a GAI tool that uses deep learning algorithms to understand natural language and generate responses to complex questions, based on the context of the conversation.
Tools like ChatGPT have significant implications for businesses and business analytics in particular. These tools provide users with an intuitive, natural language interface with their systems, making data more accessible and insight extraction more seamless than ever before. It can help them automate their customer service operations and provide personalized responses to their customers.
However, as with any new technology, there are challenges and limitations professionals must face before they can make the best use of these powerful tools. For example, while these large language models are experts in manipulating and drafting natural language text, they often struggle with mathematical calculations and factual responses. In this article, we will explore the transformative impact of AI in business analytics, with a specific focus on the capabilities and challenges posed by ChatGPT.
The Role of AI in Business Analytics: A Deep Dive
Contrary to what it may feel like in the current hype cycle, AI isn’t new. It has been around in one form or another for decades. But it’s only in recent years that sci-fi and mostly academic passion has crossed into the realm of real-world applicability. The potential of AI in business analytics in particular has begun to be realized in earnest. Today, AI is being used across a wide range of use-cases, including automation routine reporting, business data enrichment and insight extraction across the entire revenue stack, from marketing to sales and customer success.
Some of the key benefits of AI in business analytics include:
- Increased efficiency: By automating repetitive tasks and processes, AI can help businesses save time and resources.
- Enhanced customer experiences: AI-powered chatbots and virtual assistants can provide customers with fast, personalized support around the clock.
- Improved decision-making: AI-powered analytics can provide businesses with new insights and perspectives that were previously much more challenging to obtain.
- Competitive advantage: By leveraging AI to gain new insights and optimize performance, businesses gain a competitive edge over competitors relying on inefficient, manual analytics.
Real-world examples of AI applications in business that use data analytics to enhance their products and services include:
- Google's search engine, which uses AI to provide personalized search results based on user behavior and preferences.
- Facebook's News Feed, which uses AI algorithms to curate content and prioritize posts based on user engagement.
- Uber's surge pricing, which uses AI-powered data analytics to adjust prices based on demand and supply.
- Airbnb's dynamic pricing, which uses AI algorithms to optimize prices based on market demand and user behavior.
- Zillow's Zestimate, which uses AI-powered data analytics to provide accurate estimates of home values based on various factors such as location, size, and condition.
Problems with Generative AI Tools in Business Context
Generative AI tools like ChatGPT have revolutionized the way businesses approach analytics. These tools have the potential to provide valuable insights and help organizations make data-driven decisions. However, there are several challenges and limitations that need to be addressed before businesses can fully realize the benefits of these tools.
These include:
Quality of Input: The significance of posing the right business questions
One of the most significant challenges with generative AI tools is the quality of input. The output generated by these tools is only as strong as the input provided by the user. This means that businesses need to ask the right questions in order to get effective outputs from these tools. Asking vague or poorly worded questions can lead to incomplete or inaccurate answers.
Connecting Business Data Directly: The Challenge of Data Integration
Incorporating business data directly into AI-based analytics platforms is still a major challenge for most businesses. Diverse databases, sources, structures, and protocols make integration with the language models difficult. Currently many organizations resort to manually outputting the relevant information and feeding it into language models, rather than directly connecting databases to the AI.
Black Box Behavior: Lack of Transparency and Drill-Down Capabilities
AI tools often operate as "black boxes," meaning it's impossible to observe how they arrive at their conclusions. This lack of transparency can be a significant drawback for businesses that require in-depth data scrutiny and audit trails before making any meaningful business decision. Without the ability to investigate the underlying reasoning and data used by the model, businesses are left to trust the outputs blindly, which is still too risky for many decision-makers.
Calculation Limitations: Inaccuracies and risks associated with relying on ChatGPT for numerical calculations
Lastly, there are calculation limitations associated with relying on ChatGPT for numerical calculations. While it is excellent with text-based queries, it may not be accurate when it comes to numerical calculations. Relying on ChatGPT for numerical calculations can lead to potential inaccuracies and risks.
Outdated Training Data: Risk of receiving outdated or irrelevant answers
Outdated training data is also a significant challenge with generative AI tools. ChatGPT's last training data was from 2021, which means that there is a risk of receiving outdated or irrelevant answers to questions that require up-to-date information. This is particularly problematic in fast-moving industries where trends and best practices can change rapidly.
Lack of Contextual Understanding: General nature of ChatGPT's responses
Another challenge with generative AI tools is the lack of contextual understanding. These tools provide general answers that might not always cater to unique business contexts. This can lead to reduced efficiency, missed business opportunities, and limited insights from customer interactions. For example, a chatbot might provide a generic response to a customer query without taking into account the specific products or services offered by the business.
Token Limitation: Challenges posed by ChatGPT's token limit
Another challenge with generative AI tools like ChatGPT is the token limitation. ChatGPT has a token limit of 4k, which can have an adverse effect on your API and how you can use it. This limitation can lead to incomplete or inaccurate answers, particularly when dealing with complex or multi-faceted questions.
The Future of AI in Business Analytics
Despite these challenges, the future of AI in business analytics is full of promise. As the technology continues to evolve, we can expect to see more advanced GAI tools that are better able to handle complex queries and provide more reliable - and transparent - responses. Additionally, we can expect to see more businesses adopting AI-powered BI tools as a way to transform their operations and gain a competitive edge.
One area where there is a particular need for innovation is revenue analysis. While GAI tools like ChatGPT can provide valuable insights into customer behavior and market trends, they are not yet capable of providing a comprehensive revenue analysis engine. This is an area where there is enormous potential for growth and development in the coming years.
Conclusion
The integration of AI into business analytics marks a transformative era for industries worldwide. It offers an innovative lens through which data can be analyzed, interpreted, and acted upon. Pioneering tools such as ChatGPT exemplify the promising capabilities of Generative AI, granting businesses unprecedented insights and operational optimizations. However, these advancements are not without their unique set of hurdles, from ensuring quality inputs to grappling with outdated training data. Despite these challenges, the trajectory of AI in business analytics remains overwhelmingly optimistic. As we move forward, continuous adaptation and a deep understanding of both the potential and limitations of these AI tools will be paramount. By doing so, businesses are poised not only to navigate the complexities of the digital age but also to thrive within it, securing a strategic advantage in an increasingly competitive marketplace.
Talk to an expert
Learn about challenges and opportunities of AI in Business Analytics