- Building the analytics end-state helps you collect the data you need
- Being directionally correct gives you an early glimpse of trends and insights
- Early focus on analytics helps you reward cleaning and leverage data culture to your benefit
- There are cheap safeguards to ensure only clean data makes it in
Rethinking 'Garbage In, Garbage Out' - Start business analytics without perfect data
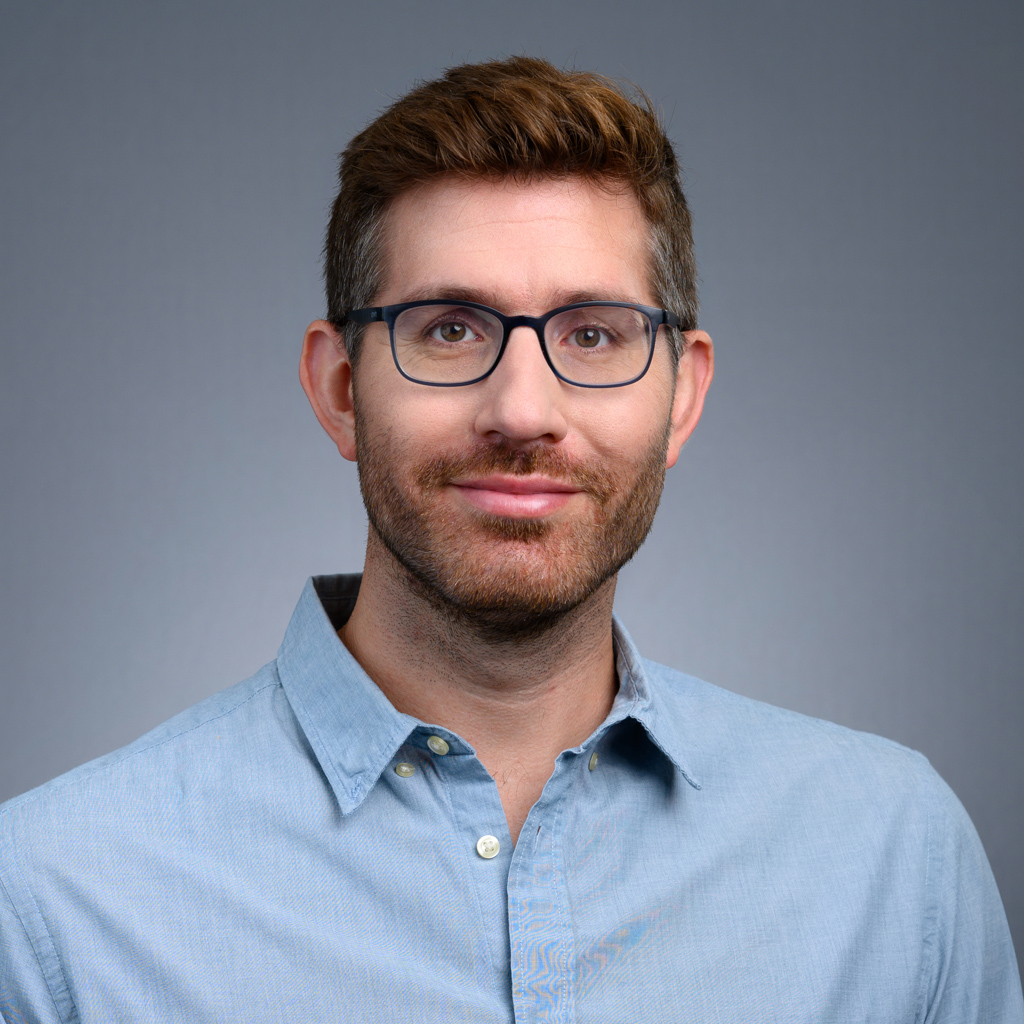
VP Data & Product Strategy
A couple of years ago, I held a webinar about how to structure your CRM to support critical SaaS metrics. I prefaced my presentation with the common idiom “garbage in, garbage out.” It’s one of those universally accepted truths that no data professional would challenge. To get effective analytics, you need to invest in your data, design the right model, and put in place guardrails and tests to ensure sanity (full, clean, and accurate data).
Clickbait title notwithstanding, I’m not here to challenge this principle. However, I do want to highlight an unintended consequence of the “garbage in, garbage out” purity. This notion often leads teams to employ a waterfall approach, delaying implementing analytics until their data is perfect. Let me share a secret: your data will never be perfect.
If you find yourself saying, “I’m not ready to start building my business analytics because my data is not perfect,” I’d suggest a few additional perspectives to consider.
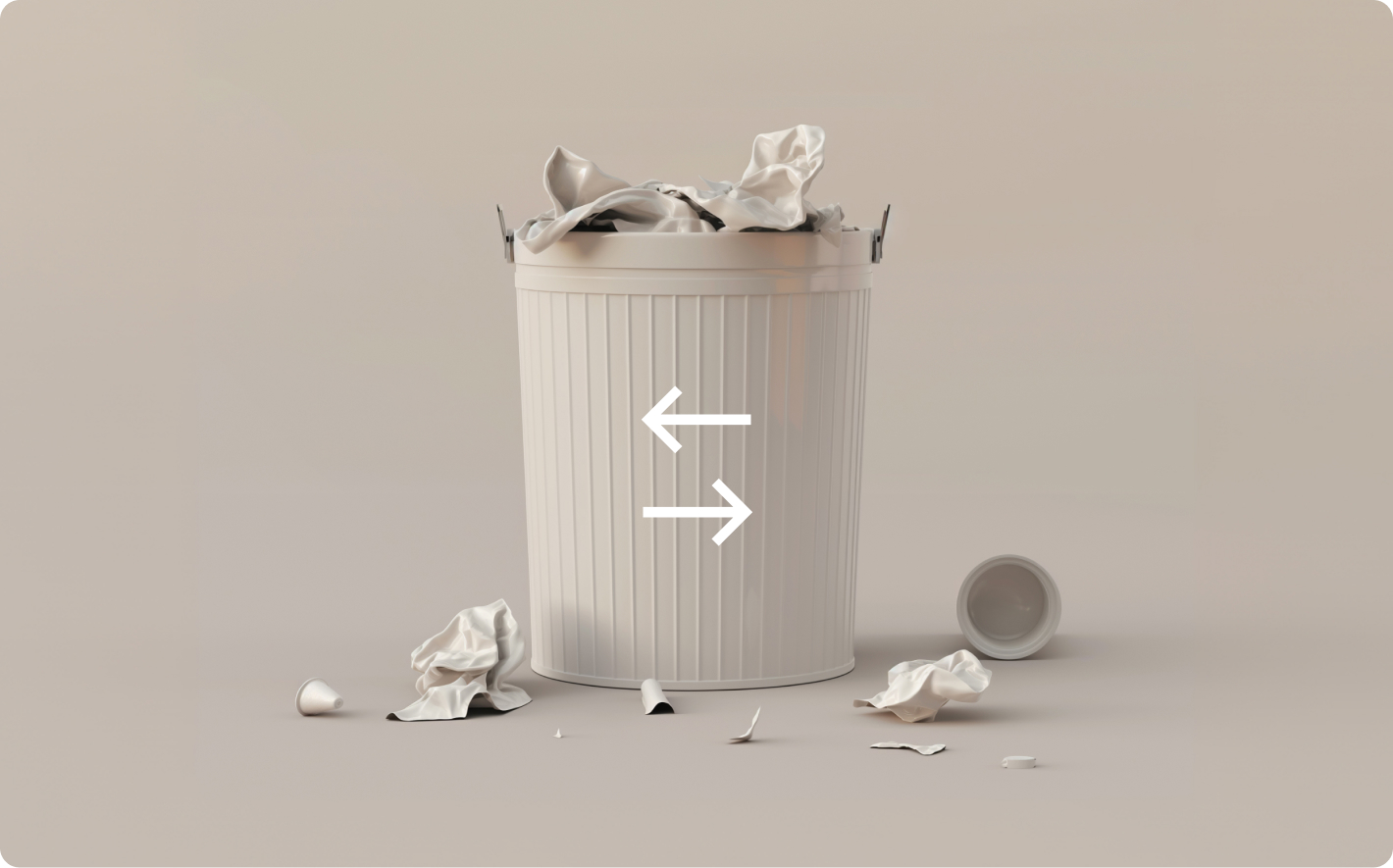
Building the analytics end-state helps you collect the data you need
It's very difficult to design a data model a-priori, planning what you’ll need for the analytics use cases you’re interested in. It’s easy to spend significant time and resources on a data model that misses some critical pieces and collects other pieces that turn out to be unnecessary.
Even if you think of the general use cases you’re going to look at, the devil is always—and I mean always—in the details (“did I turn on field tracking in Salesforce for this data point?”). By envisioning the analytics end-state, you can create a roadmap that guides your data collection efforts.
However, it’s not enough to plan just on paper. When you start building the analytics - transformations, calculations, visualizations, etc. - even on a limited sample, you quickly discover what you actually need. This hands-on approach helps identify gaps and critical data points that theoretical planning might miss. It ensures your data model is practical and aligned with real-world requirements, saving significant time and effort in the long run.
Being directionally correct gives you an early glimpse of trends and insights
Perfect is the enemy of good, and often getting 80% of the data right can provide you with fairly sound, directionally correct answers to your immediate business questions.
Ideally, aim to approach this in defined increments. For example, clean the data segment by segment or region by region. This way, you can push to your analytics engine only those segments of the data that have been cleaned and which you can trust. You gain earlier access to something to work with.
Naturally, you need to take this data and insight in the relevant context, understanding what’s in and what’s still missing. But if you treat it appropriately, it can be a powerful tool—much earlier than you’d otherwise get it.
Interested in transforming your analytics approach?
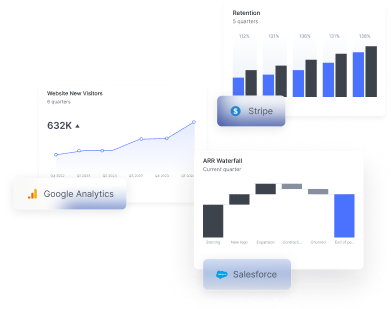
Early focus on analytics helps you reward cleaning and leverage data culture to your benefit
Having standing analytics deliverables (in the form of dashboards, reports, and GenAI interactions) to which new data is constantly added starts to build an organizational culture centered around data. It draws executives’ attention to which data is cleaned and which isn’t, incentivizes teams to clean their data, and provides “instant” gratification for cleaning your data.
If you work in a waterfall method, waiting to build analytics only when 100% of the data has been verified, people who put in the work now will only reap the rewards at some point in the future. Instead, if verified data feeds directly into high-visibility analytics deliverables, the gratification is instant.
You have, in effect, gamified data cleaning. And the impact can be drastic.
There are cheap safeguards to ensure only clean data makes it in
Small tricks, from adding a field: Verified: True to filtering the visualization to include only approved segments; there are cheap, easy-to-implement safeguards to make sure that only clean data makes it to stakeholders. These do not replace effective safeguards at the source, which are diverse and important (validation rules, mandatory fields, standardization, duplication checks, outliers, and anomaly detections, among others). Instead, these small tricks are designed to accompany the transition period of cleaning the data, allowing you to make analytics available even during the transition.
---
By understanding that “garbage in, garbage out” doesn’t have to mean a waterfall approach to analytics, you can start leveraging your data much earlier in the process. This approach not only accelerates the delivery of insights but also fosters a data-driven culture.
Interested in transforming your analytics approach? Contact us to learn more about how Sightfull can help you achieve your analytics goals.
Don’t Wait for Perfect Data
Book a Demo to Leverage Analytics Without Delay!
Precisely track and analyze your revenue performance
Gain access to essential insights and trends that directly impact your ability to drive revenue growth and enhance business performance.